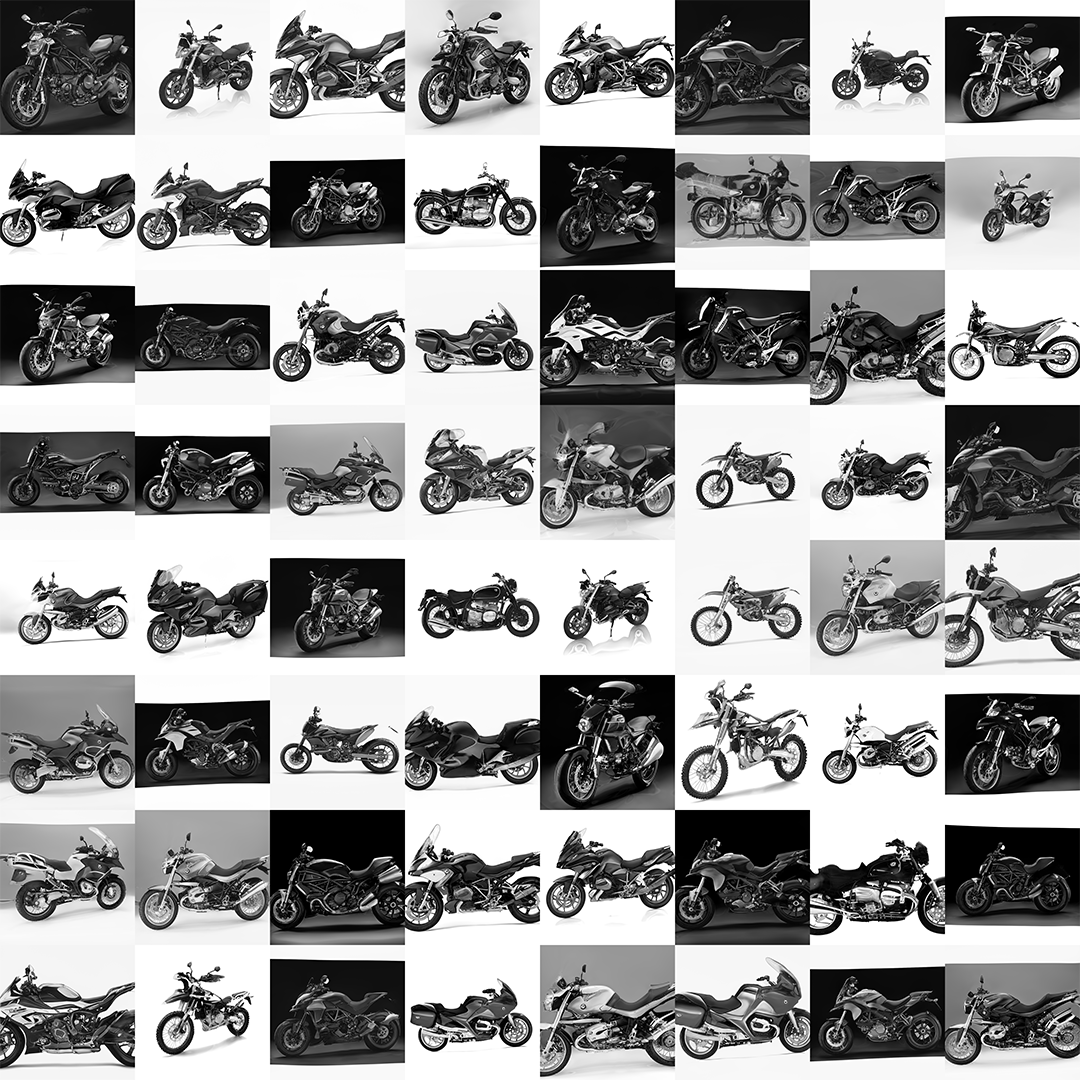
MotoGAN
2023
Digital & Interactive
Collaboration with Onur Sönmez
Embarking upon a curious expedition into the realm of transportation design, this AI-driven machine learning project titled Motogan (Motorrad Generative Adversarial Network) seeks to unravel the enigmatic essence of what constitutes a quintessential BMW motorcycle. By delving into the intricate subtleties and unique features that set various designs apart, Motogan aims to synthesize a wide range of hybrid motorcycle model images, drawing inspiration from diverse, iconic brands such as Yamaha, KTM, Ducati, and Kawasaki.
Motogan helps us, human designers envision an experimental landscape where disparate components are meticulously grafted from one motorcycle to another, breeding a multitude of hybrids that exhibit unprecedented aesthetic and functional prowess. The machine learning-based images it generates traverse conventional design’s boundaries. Morphologies of these speculative vehicles are shaped by the inventive interplay between the innate traits of currently existing motorcycle models. The dynamic synthesis model that Motogan operates on yields an evolutionary tree of images, blossoming with an array of vibrant branches that represent the countless possibilities forged by the harmonious melding of disparate design philosophies.
As with any experiment in the laboratory of creative work, not all hybrid models appear to be impeccable. Some bear the burden of excessive mutation, their designs rendered desirable for a whimsical designer’s imagination. Yet, this element of risk and uncertainty enriches the machinic output of Motogan with an exhilarating sense of discovery. This exploratory project serves as a testament to the boundless potential of AI-driven machine learning by melding the inimitable allure of BMW motorcycles with the distinctive design elements of other esteemed brands. Ultimately, Motogan strives to generate a cornucopia of innovative models that challenge the commands of convention and exceed the limits of our present-day imaginations for reshaping the very fabric of transportation design.
In Collaboration with Onur Sönmez.
Project Text: Mehmet Ekinci
Technical notes:
Custom Stylegan3 model trained on a dataset of grayscale images. The dataset consists of a core dataset which is used with a center crop and extended to be squared, trained with x-flips on 4-6 RTX2080 TI's running CUDA 11.3.
Since the 2080 TI has a pretty awkward VRAM size of 11GB the model was trained in batchsizes of 16 using pytorch 1.10.1
The training took place over the course of 7 days.
Even though Stylegan3 can theoretically run in realtime on an single instance - threaded even multiple, the mosaic projection on the LED Wall consits of individual, prerendered latentwalks composed and mapped with Touchdesigner. The multi video loader is fully customizable, parameterized and handles realtime changes to layout, quetimes and videocount.
.tox to come!
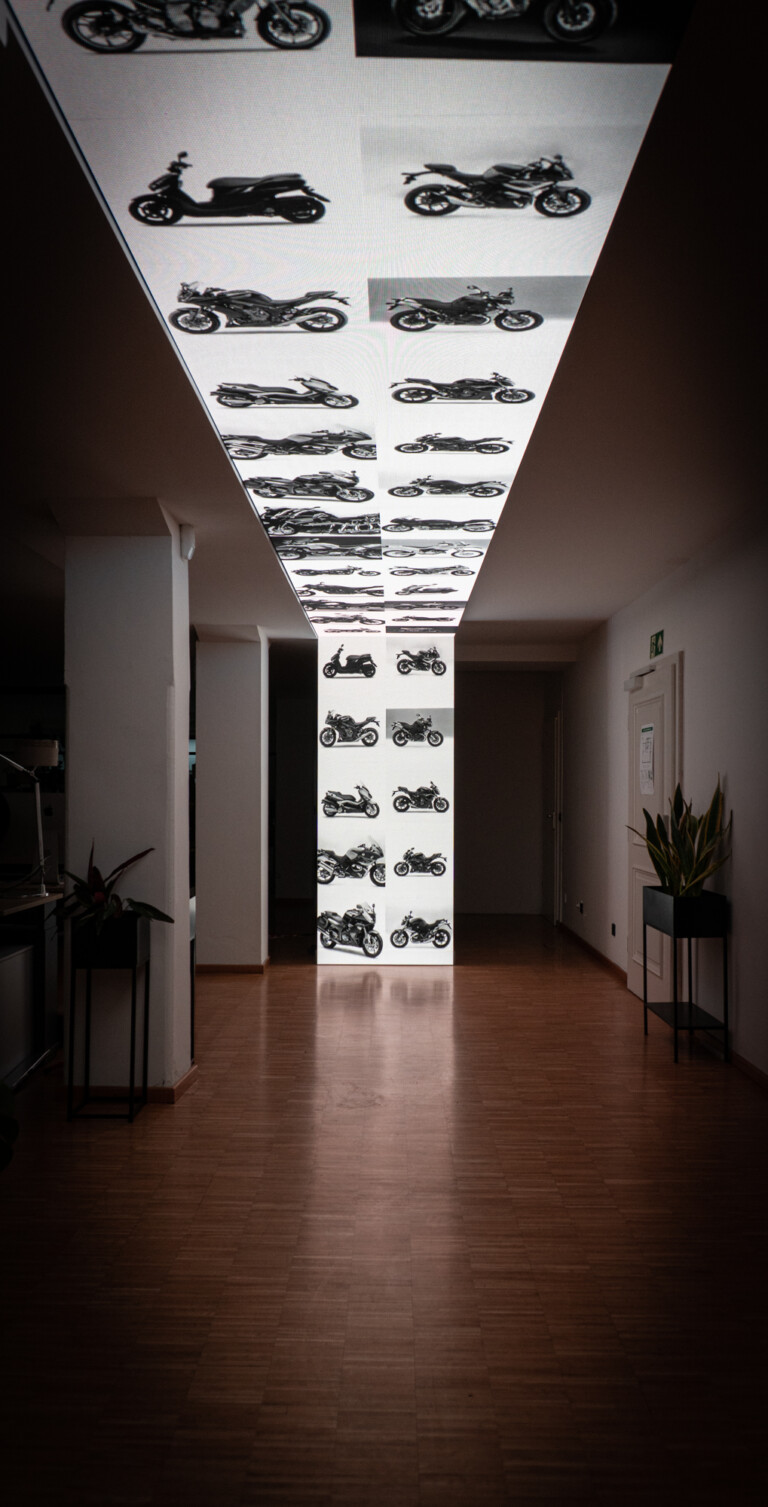
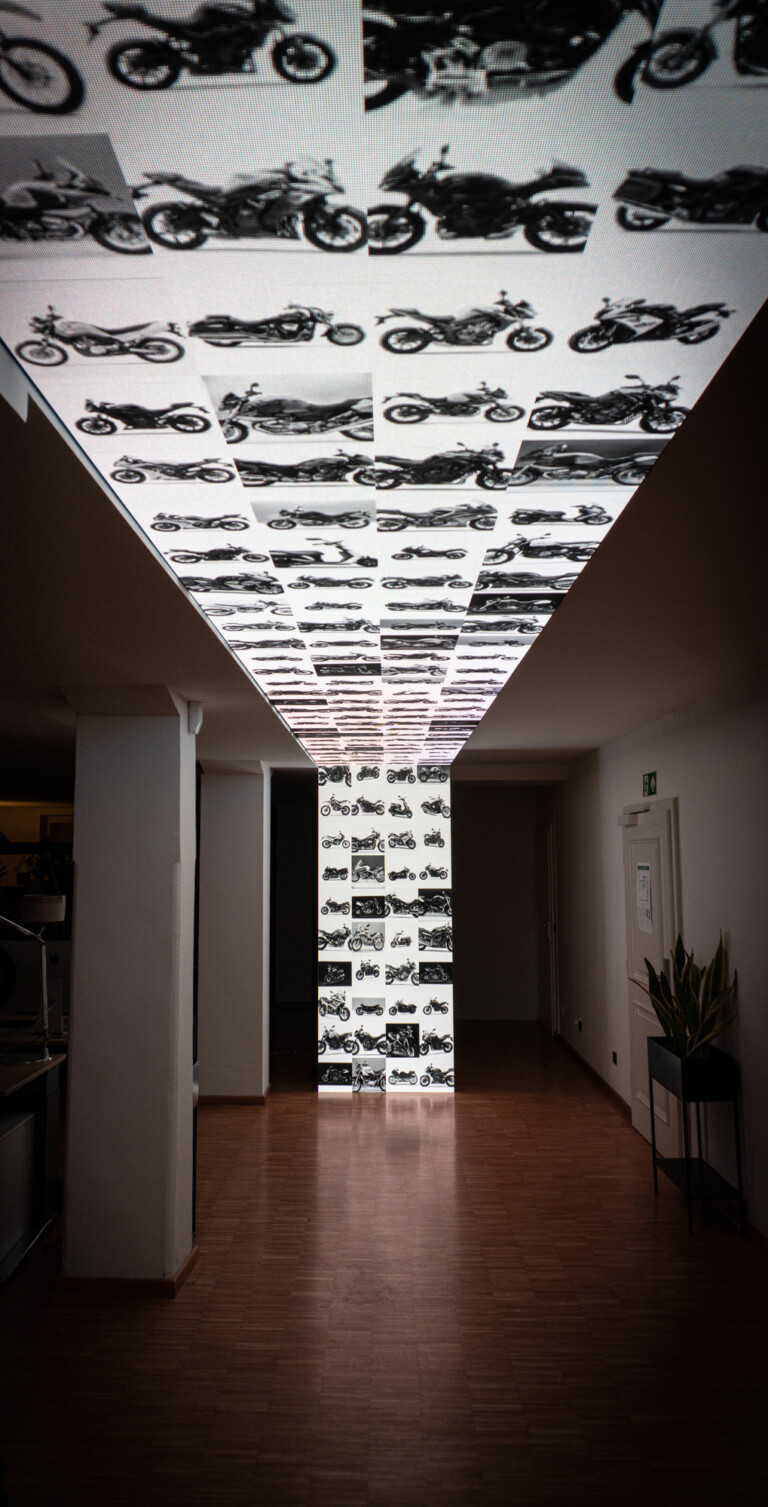
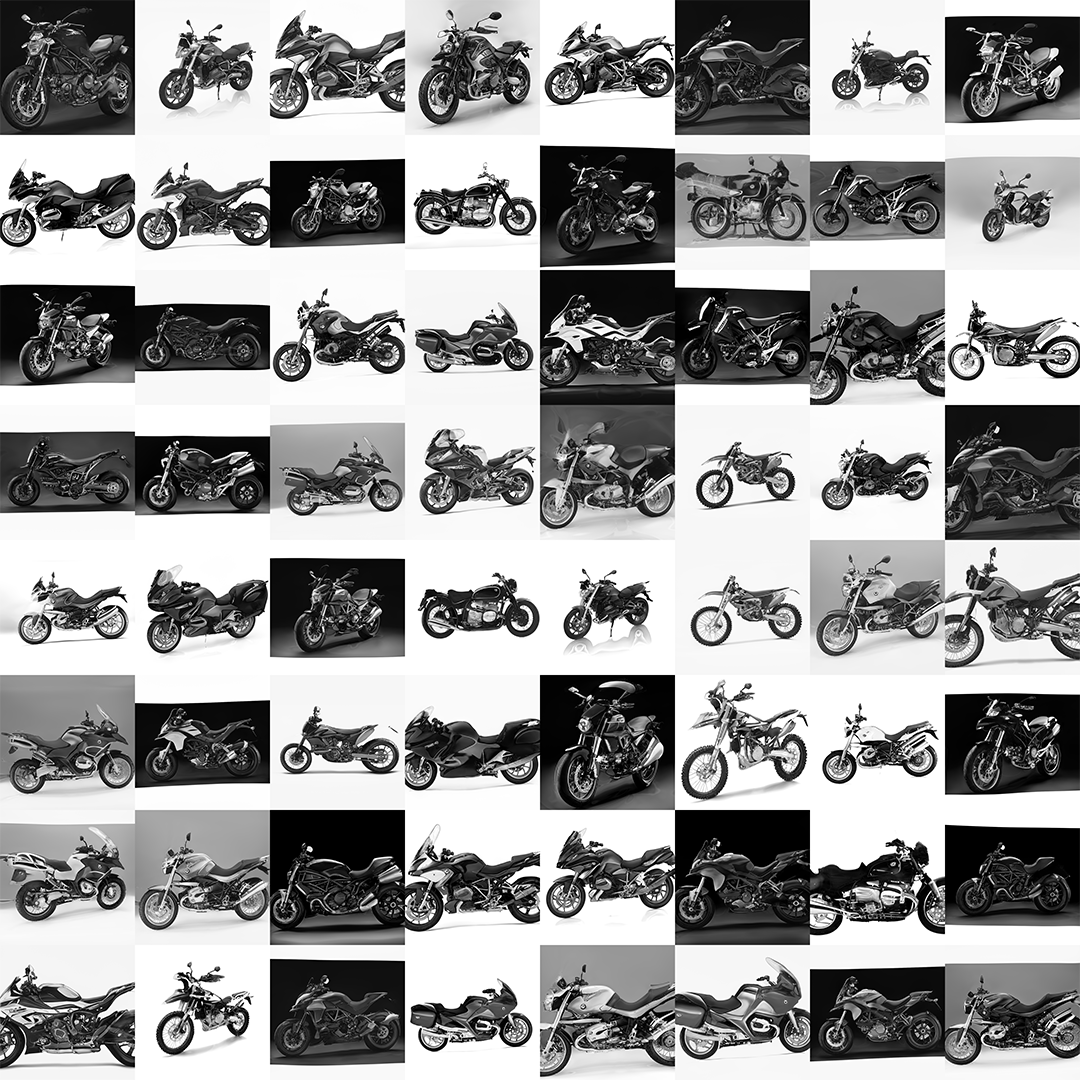